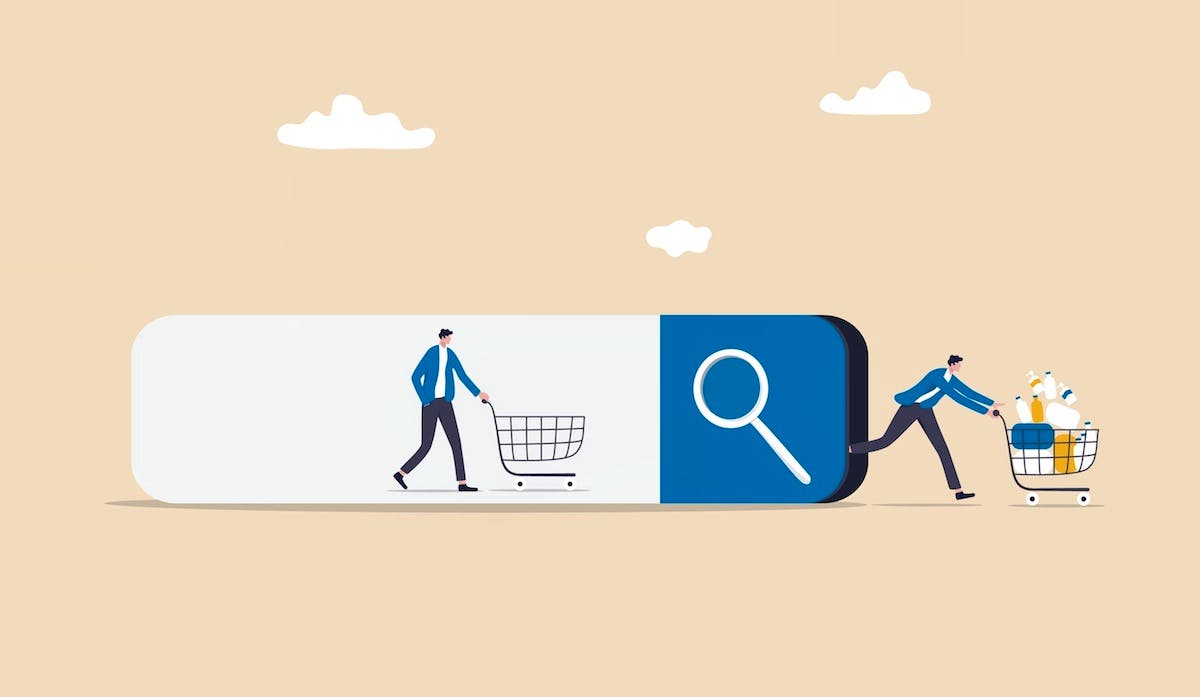
We’ve seen lots of examples of new intelligent search features or conversational agents in the last six months in online retail – from Walmart, Instacart and Amazon, for example.
In a recent article, I tried out some AI-powered product discovery features to see how useful they might be for shoppers. And though they each provided distinctive experiences, and AI-powered search is fairly obvious when you use it (it’s often labelled as such), I was left with the uneasy feeling that I wasn’t sure exactly how to define it.
So, what exactly is AI-powered search and what does it mean for retailers?
The short answer is that advances in machine learning now enable deeper understanding of:
- your search query (and you, or your browsing behaviour);
- a retailer’s product catalogue;
- and which results might prove popular.
This deeper understanding, along with the option of using a large language model (LLM) to generate responses, opens up many possibilities for retailers, to offer greater inspiration for customers that don’t have the time or inclination for product discovery and research.
And, of course, retailers are very happy to adopt this new technology. We know that customers are drawn to the search bar, and that search hasn’t always been particularly effective when it comes to the so-called long tail (queries that tend to be longer, more nuanced and seen less often, if ever). Therefore, improving search is potentially an incredibly cost-effective way to increase conversion on your site or app.
There’s also an additional automation benefit, with search requiring less manual correction when backed by new AI models.
Hasn’t search been AI-powered for some time?
Search engines have been smart for a decade (this wouldn’t be an article about AI in marketing if it didn’t make the point that AI is actually nothing new). The Hummingbird algorithm is often talked about as the death knell for keyword-stuffing tactics and the beginning of semantic search, but Google refers to Rankbrain as its first AI system for ranking that helped to pin words to concepts. In 2018, Google began using neural networks to better understand how queries relate to pages, and 2019 saw a big leap forward with BERT, allowing for better understanding of how “combinations of words express different meanings and intents”.
Of course, all the talk of the past two years has been about the rapid progress in LLMs (such as Google’s Gemini) that have brought us to the point where we can talk to a chatbot or the search bar as if it were a personal assistant – both in some search engines (such as Copilot in Bing) and on some brand apps and websites.
Gemini 1.5 Pro, Google’s latest generative AI model, has a larger ‘context window’ than previous models. It can process around 700,000 words of context, but is also multimodal, capable of processing video and audio, and can keep abreast of a conversation (with a shopper and that shopper’s preferences and history, for example). The model is better able to understand the “narrative flow of data”, then generate “contextually richer responses” and “reduce the need for fine-tuning and factual grounding”, as Techcrunch explains. LLMs can also, for example, open up the possibility of multilingual searches on a website, allowing customers to search effectively in Spanish, on a site using English product descriptions.
Google has only just this month rolled out some AI-generated search experiences to the UK. There’s an ongoing debate about the ethics of using LLMs to generate answers to search queries (rather than returning a list of URLs), given the potential for hallucinations, bias, copyright issues or simply publishers losing traffic. Indeed, many have suggested that Google’s rush to market in the wake of ChatGPT’s partnership with Microsoft has been partly responsible for high-profile missteps such as inaccuracies in Gemini’s image generation of people, and incorrect information shared by its chatbot, then called Bard, in early 2023.
Will Search Generative Experience (SGE) really transform web search?
In this article, we’re focusing on product searches in retail, and though the sector is not immune to these problems, many AI-powered ecommerce search experiences are much narrower in their output, and often still focus on returning discrete lists of products.
The evolution of commerce search
Google’s Vertex AI Search was launched in summer 2023 and allows enterprise customers to build intelligent search experiences utilising the Gemini model. Vertex can be used as a RAG (retrieval augmented generation) system, which powers generative AI applications by combining retrieval and generation models i.e. supplementing an LLM’s knowledge base with your own business context.
At the beginning of this year ahead of NRF2024, Google Cloud announced its solution specifically for the retail vertical – conversational commerce – based on this technology. Crucially, businesses can now deploy virtual agents in “a matter of weeks”, to assist shoppers and provide product recommendations based on their preferences. Retailers can also adopt “Google-quality search, browse, and recommendations natively embedded on their digital storefronts” and will be able to “custom-tune a LLM to their unique product catalog and shopper search patterns, which can dramatically improve their ability to surface relevant products to customer queries by better ranking potential products as a fit for any given search term.”
Of course, Google is by no means the only solution available to create these kind of experiences. Walmart has worked with the Microsoft Azure OpenAI Service, for example (as well as developing its own models), and there’s a burgeoning market specifically in commerce search, with companies such as Coveo and Algolia.
The Q3 2023 Forrester Wave report for commerce search makes the point that many online retailers have traditionally used search functionality that came bundled with a commerce platform, but that the market is shifting towards standalone solutions in commerce. Twenty one of 27 retailers interviewed by Forrester were using their first packaged commerce search product.
And the big platforms are responding to this desire for improved search. Shopify introduced ‘Semantic Search’ in January of this year, urging merchants to “Drive sales and increase product discovery with this new search feature that goes beyond keyword matching and understands buyers’ intent, returning richer and more relevant results.”
Explaining semantic search, vectorisation, and the move away from exact keyword matching
Most of the commerce search companies offer blog posts or landing pages explaining how AI-powered search works in layman’s terms (I found Algolia’s simple guide to be pretty helpful).
The old style of search on an ecommerce site (the type we discussed as struggling with the long tail) was all about query-keyword matching. This type of search is fast when the answer is there – think searching for ‘socks’ on an apparel site – but can’t cope with more complex queries.
This is why machine learning has, for a number of years, been brought to bear on the three stages of a search:
- query processing – where semantic understanding is sought.
- retrieval – finding matching items, such as SKUs, which are relevant to the query.
- ranking – ranking retrieved results based on relevance to the searcher and their query, and applying reinforcement learning to continually optimise as shopper preferences, retailer objectives and their product catalogues change.
We have referred to semantic search already. It is one element of AI-powered search, and it offers better understanding of the meaning of a query, though the use of natural language processing to understand related and relevant concepts, including synonyms.
Vector search, as Google puts it, “unlocks powerful semantic matching”. Vectorisation is a process of creating a numerical representation (embedding) of items and words in order to map how closely they are related. Retailers can build an index from their embeddings, which can then be deployed for querying.
As Algolia details, vectors can be large in size, and therefore difficult to work with (neural networks are now being used to compress vectors to allow for quicker processing), and combining keyword and vector results is hard to do (with vectors used as a ‘fallback’ solution in the past).
Applying machine learning across each stage of the search means ecommerce businesses stand to benefit in multiple ways, with improvement over time.
So, what does AI-powered search mean for the ecommerce experience?
For online retailers there are many ways in which product discovery can become more sophisticated and ultimately assist the shopper:
- Shoppers can actively ask for inspiration: ‘How should I complete an outfit?’ ‘What goes well with seabass?’ ‘Show me everything I need for a movie night.’
- Dynamic merchandising: filters and assortment can change depending on the search query, with filter chips added to faceted search, for example.
- Getting rid of faceted search altogether in some cases: See for example Rodo, the automotive marketplace, which will show 2 or 3 results and take the hassle out of finding the right make and model.
- Personalised search results updated in real-time: This may not be obvious to the user, but can include making assumptions about what the shopper is in market for, and ranking these results higher.
- Improvements to predictive search: trending products, for example, are easily identified and can be prompted.
- Virtual agents that can streamline the process of finding and buying: Think about the shopper on Amazon, for example, who is in a hurry and wants to find a fairly inexpensive but reliable product in electronics (e.g. headphones or an LED light) but doesn’t want to wade through reviews, scroll down looking for Amazon’s bestsellers etc. This is the sort of long-tail query that can be catered to (the implications for the ad model are another discussion point).
Walmart is probably the retailer that is getting the most press coverage for this technology at the moment. In an earnings call in February, CEO Doug McMillon said, “The thing we’re most excited about that’s already happened is the way search has improved, and the way generative AI helped us really improve a solution-oriented search experience for customers and members. And it happened pretty quickly.”
Walmart plans to offer the shopper a single place to, for example, plan an event, and its progress is a potential anxiety for Google. Insider Intelligence’s Jacob Bourne told CNBC that with AI-powered search becoming “a norm for online retailers”, the question is whether this will be “a death by a thousand cuts for Google Search”.
Of course, Google hasn’t had its own way in retail for quite some time, given that Amazon is many people’s first point of call for product searches. And Google is also implementing its own new features in its search engine, with Shop with Google AI allowing users to generate images and then select the one they like, before Google heads off and retrieves similar looking products.
It’s hard to predict the future of product discovery in retail, given that the more conversational experiences made possible by generative AI seem best suited to large and broad product catalogues, for the likes of Walmart and Amazon (NB. Rufus, Amazon’s assistant, was this month seen handing out coding advice, so it will be intriguing to see how its scope and that of other virtual agents is defined).
For considered purchases such as vehicles and holidays, generative AI solutions seem like a neat alternative for shoppers bored of traversing faceted search, review platforms, third-party publishers, blogs and so on.
And if the baseline achieved in the next year or so is more ecommerce sites with more useful semantic search that converts, that would seem to suit shopper and retailer.
Gone are the days when knowing ‘how to search’ was the mark of a savvy web user.
If you’re looking for training, explore Econsultancy’s AI in Marketing short course, ecommerce and CX training, bespoke academies and skills benchmarking.
Comments